Not good – buyer beware as the results were inconsistent textually and from a performance perspective, where it suggested index trackers (without specifying Indices) and otherwise generally poor performing funds. This is not surprising[1] as it is akin to asking an English major to solve a differential equation – pun intended! The math here is in ingesting existing published results and making them contextually available and not on training the models to accurately select the asset. As such, the basis of ChatGPT is the Large Language Models (LLM) that are trained on existing ‘outcomes’ that are solicited from the training information and this makes them both a good and a bad candidate for disseminated information including,
Good
- Moving from search queries giving links to textual results that are synthesized based on previously experienced consumption and similar suggestions
- Well suited for standardizing language or boxed results (e.g., disclosures, context, etc)
- Removing the drudgery of mundane tasks such as collating information
- Offering premium shelf space for willing sponsors
Bad
- Curating the textual results from the training set of information (potentially itself curated or influenced) with a potential to manage the narrative[2]
- Randomizing outcomes or based on algorithm priority (e.g., frequency, region, sponsored, etc)
- Hedging results via the disclosures, but giving interpretation/inference (e.g., best, popular, etc) as if it has ranking (vs. listing) models
- Amplifying self reporting or similar biases from ingested datasets
- Presenting results that are sponsored or biased, without disclosures
Overall, the use of the LLM models here is more like crowdsourcing the recommendation, where at a minimum back testing (and simulation) models should be added to make the results disclosure appropriate. Further, for improving the selection, performance ranking model (RM) results would need to be added as a feed to the LLM models. This is already being done locally via Natural Language Processing (NLP) models connected to the ranking models assessing the whole dataset, clusters, regimes, etc (note that this is the underpinning of the Ask2.ai platform). These aspects were covered in our Insights piece – Is Artificial Intelligence deployment the new Y2K? Where we show how selecting financial assets largely lies in the non-stationary quadrant, where degrees of continual tuning and expertise is necessary.
ChatGPT “recommendation” is akin to googling fund suggestions, but the textual delivery makes it more relatable (and potentially comforting for the less discerning user). This makes its application ripe for misuse when the selection is from over 7,000 mutual fund options (in the US alone)[3]. ChatGPT’s traditional valley get an MVP out there playbook seems to have generated the buzz, but here it seems to be treading on a regulatory minefield.
DATA
We queried ChatGPT with ‘Best US mutual fund’ and the subcategory of ‘Best large cap US mutual fund’ using mobile and desktop (over a few days). We chose the word ‘best’ because it builds in a ranking need for classifying ‘which’ of the fund should be presented. Given ChatGPT’s LLM underpinning we felt that the more pedantic ‘how’ would be more generically covered, but difficult to evaluate. Note as an extension the ‘how’ could also have a suggestion on ‘a’ method versus the ‘best’ method. Interestingly see 5 below, where it gave a ‘how’ result for the same query.
1.Best Mutual Fund (query on March 12th, 2023)
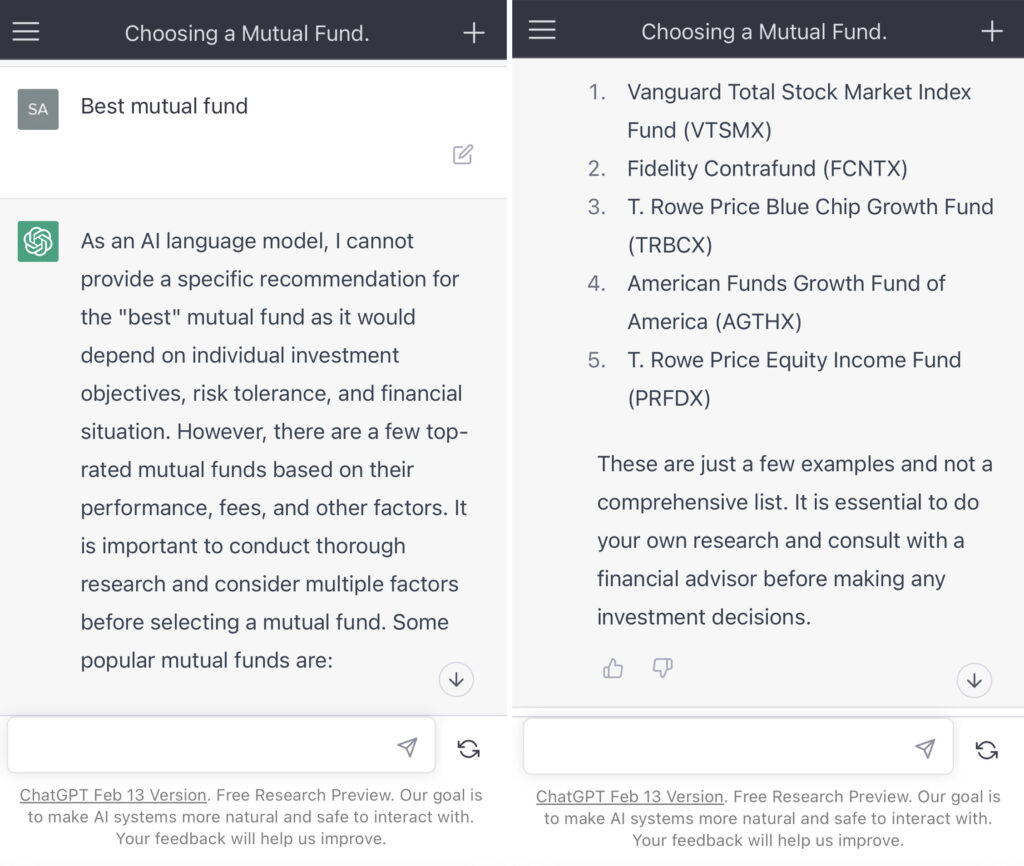
2.Best large cap US mutual fund (query on March 12th, 2023)
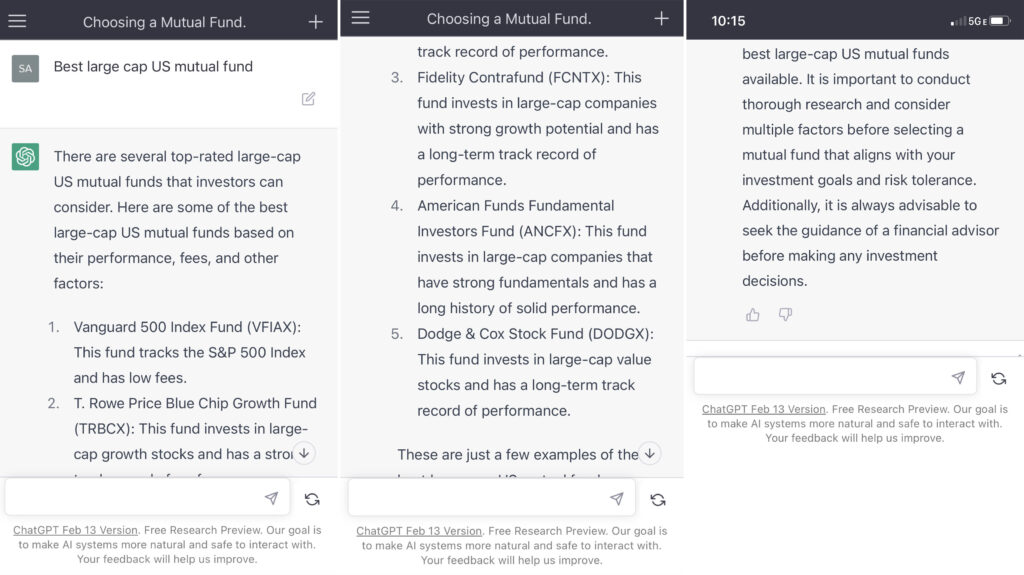
3.Best large cap US mutual fund (query on March 13th, 2023)
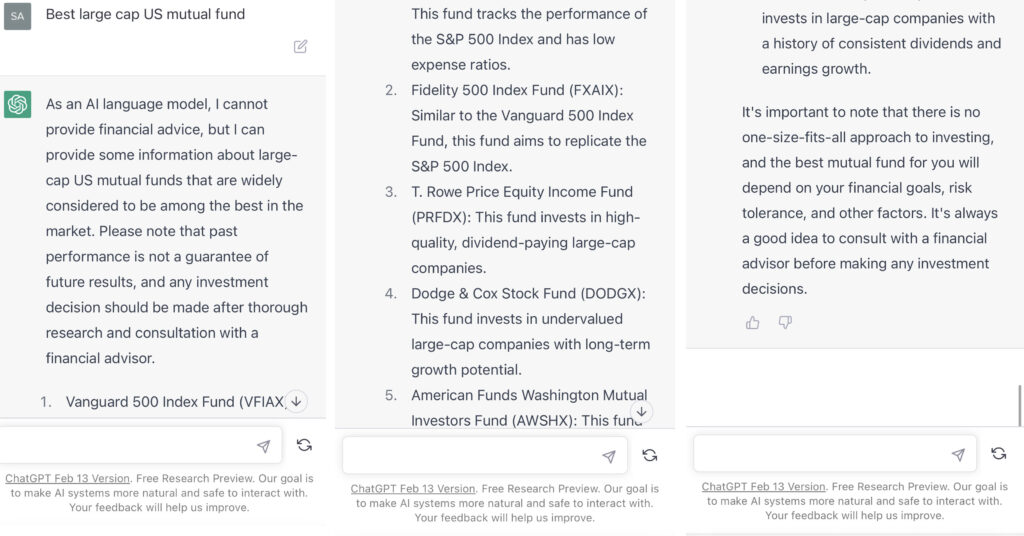
4. Best large cap US mutual fund (desktop query on March 13th, 2023)
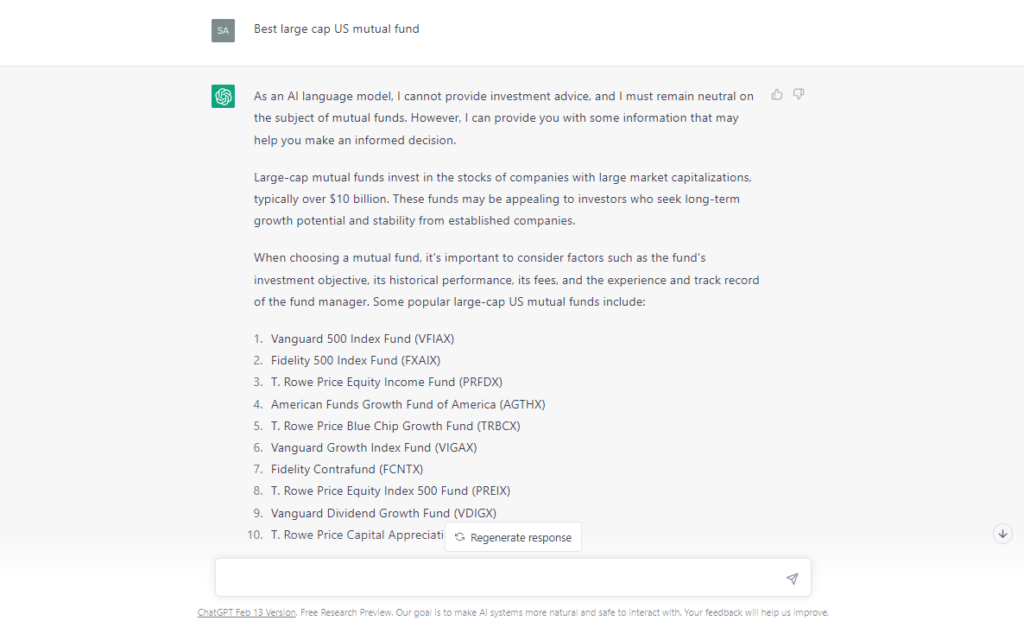
5.Best large cap US mutual fund (query on March 16th, 2023)
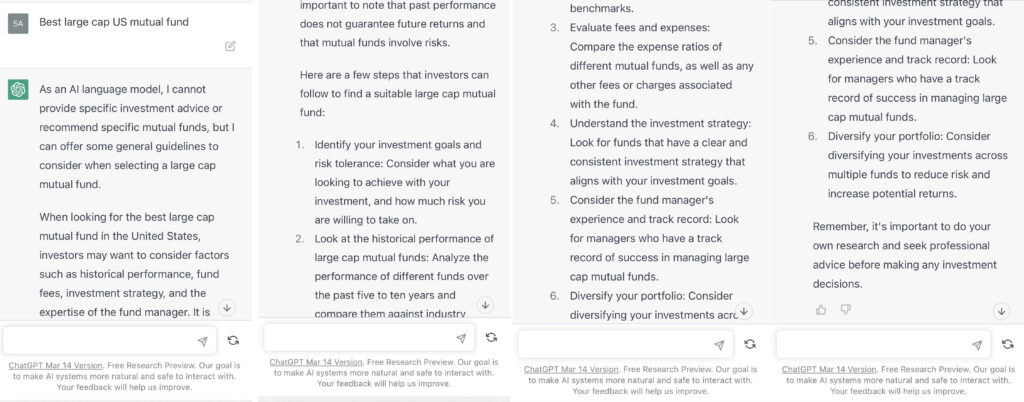
ANALYSIS
We look at the Performance Measures, Explainability Index (EI)[4] and Risk of Target (RoT) of the suggested mutual funds with data over the 12/31/2015 – 12/31/2022 evaluation period.
Textual
Results on the same query gave different disclosures and textual context, where we believe most iterations of the query would probably fail the suitability or fiduciary test and so is treading on a fine regulatory line.
1.Best Mutual Fund (mobile query on March 12th, 2023)
- Took pause to “best” due to objectives, risk tolerance and financial situation. However, gave “top-rated” and “popular” ones in any case. Based on performance, fees and “other” factors.
- No disclosure on historic performance or why these were shown. Statement on consulting a financial advisor.
- Gave 5 suggestions
2.Best large cap US mutual fund (mobile query on March 12th, 2023)
- Gave “top-rated” and “best” …based on performance, fees and “other” factors.
- Did not describe large cap
- No disclosure on historic performance or why these were shown. Statement on consulting a financial advisor.
- Gave 5 suggestions
3.Best large cap US mutual fund (mobile query on March 13th, 2023)
- “…widely considered to be among the best…”
- Did not describe large cap
- Put in historic performance disclosure. Statement on consulting a financial advisor.
- Gave 5 suggestions
4. Best large cap US mutual fund (desktop query on March 13th, 2023)
- “…I must remain neutral…” but …“Some popular…”
- Described large cap
- General statement on consideration factors. No statement on consulting a financial advisor.
- Gave 10 suggestions
5. Best large cap US mutual fund (mobile query on March 16th, 2023)
- Only ‘how’ to and no fund suggestions
Performance
Results on the same query (without any benchmark or objective function) gave different fund suggestions that were a mix of index trackers and those focused on sub categories of Large Cap Blend, Growth and Value. Even a general mutual fund suggestion query gave US large cap funds as suggestions. Assuming S&P 500 as the benchmark the suggestions (other than the index trackers) were mostly questionable.
1.Best Mutual Fund (query on March 12th, 2023)
Our query was quite generic, where
- Location: No location was specified
- Asset Class: No asset class was specified
- Benchmark: None was specified.
As noted in Table 1. and Illustrations 1. & 2., for the generic query ChatGPT did not mention a Benchmark, suggested all US Large Cap funds and further the funds were from Blend, Growth and Value sub-classifications with expense ratios ranging from 0.14% – 0.85%. For our analysis, we assumed S&P 500 as the benchmark and most of the suggested funds are questionable choices.
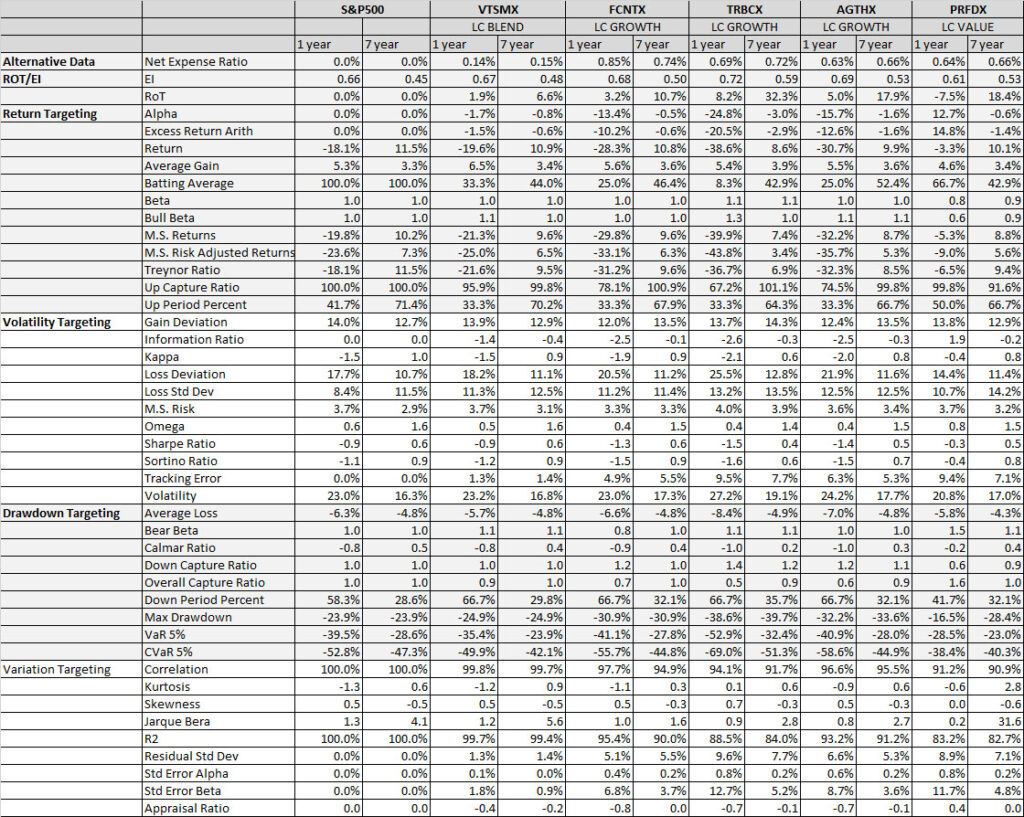
Illustrations 1. & 2., shows that on an equally weighted risk-return categories basis, only one fund (PRFDX) performs better than the benchmark, but only on a 1-year basis (on a 7-year basis the RoT is high). Another fund (VTSMX) is a lower-cost Index tracker, which could be an alternative if a broad market blend is the objective like the S&P 500.
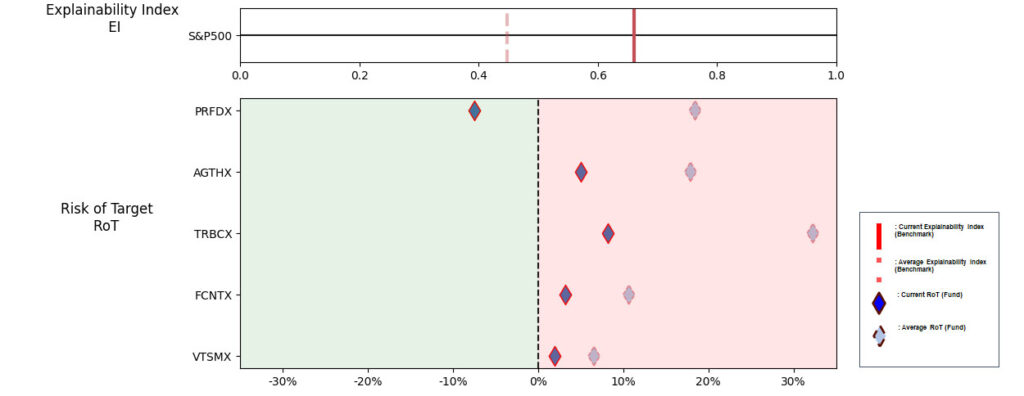
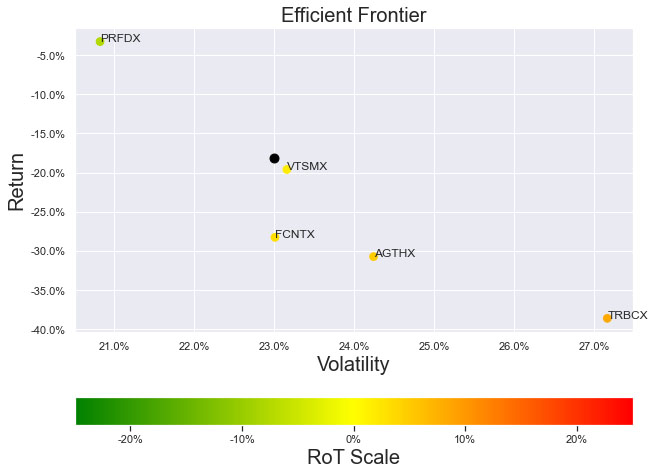
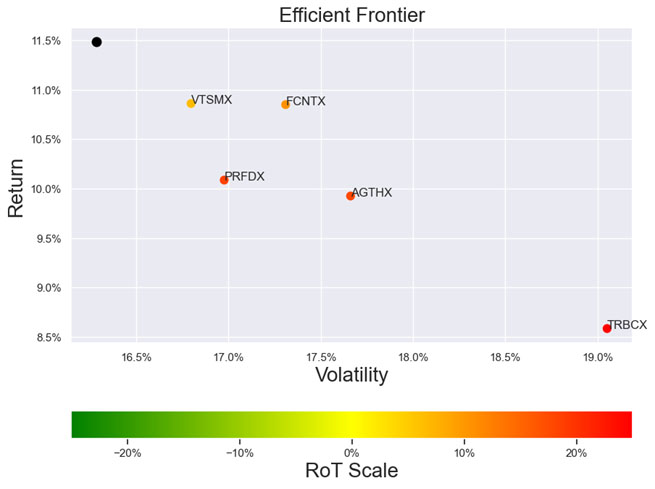
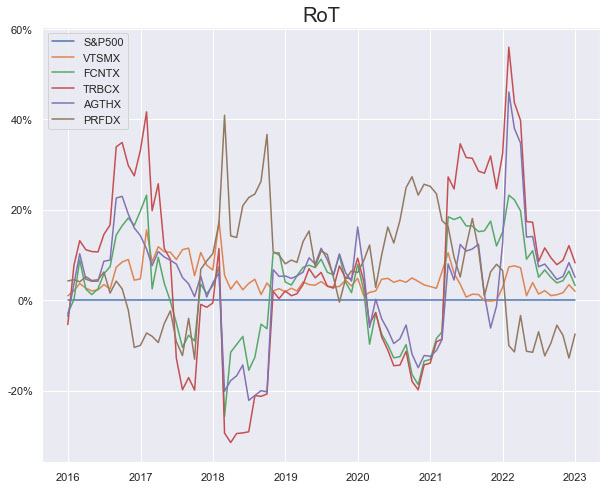
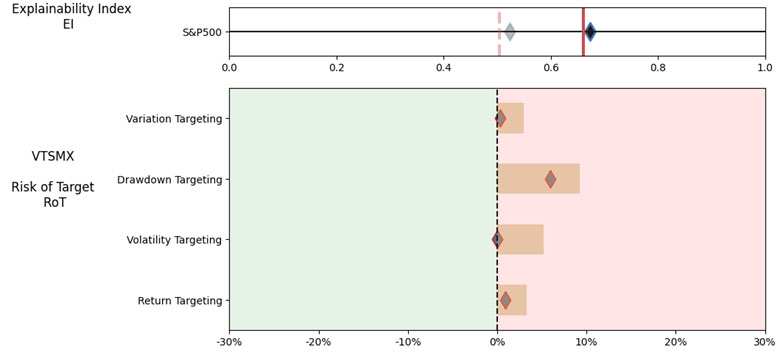
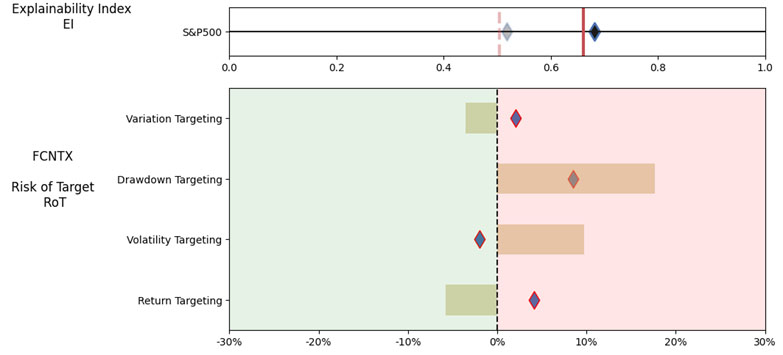
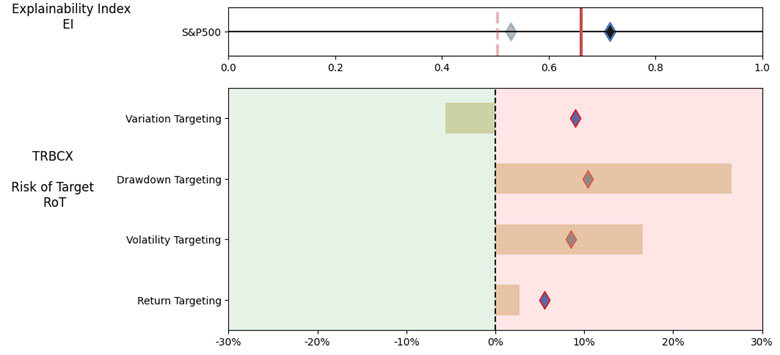
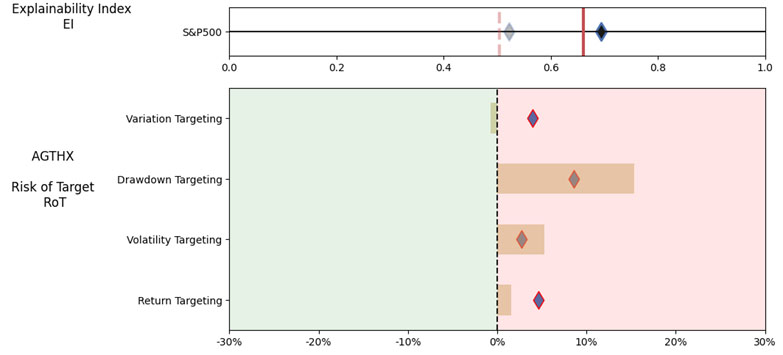
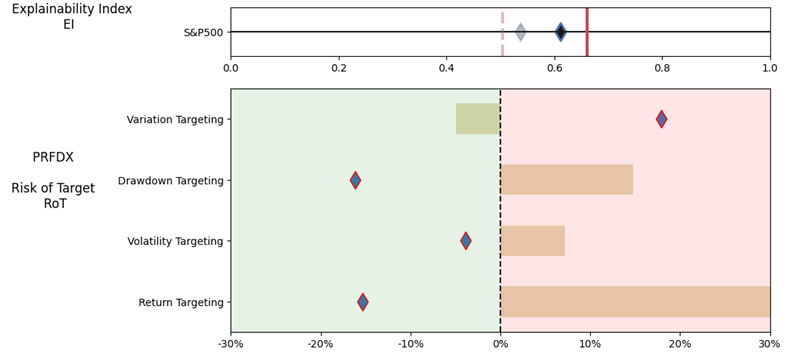
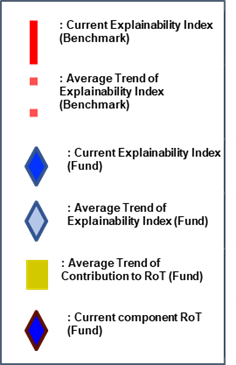
2.Best large cap US mutual fund (query on March 12th, 2023)
Our query was quite generic, where
- Location: US was specified
- Asset Class: Large Cap was specified
- Benchmark: None was specified.
As noted in Table 2. and Illustration 3. & 4., for the query ChatGPT suggested all US Large Cap funds and further the funds were from Blend, Growth and Value sub-classifications with expense ratios ranging from 0.04% – 0.85. For our analysis, we assumed S&P 500 as the benchmark and most of the suggested funds are questionable choices.
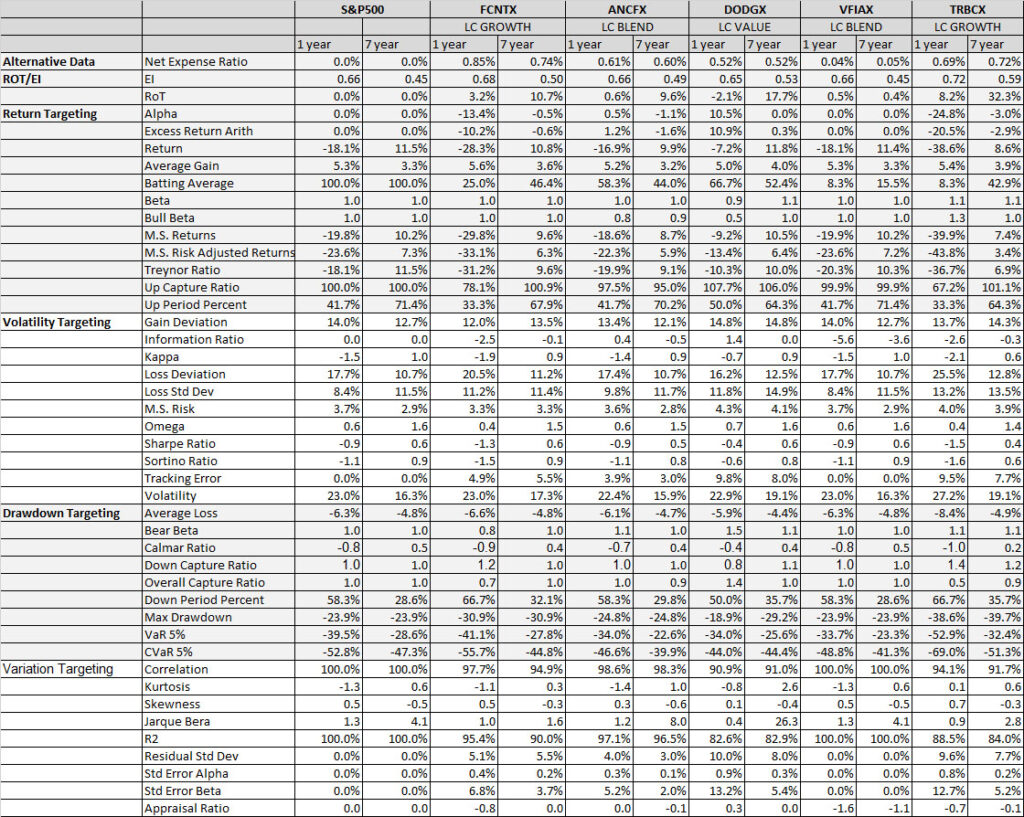
Illustration 2 shows that on an equally weighted risk-return categories basis, only one fund (DODGX) performs better than the benchmark, but only on a 1-year basis (on a 7-year basis the RoT is high). Another fund (VFIAX) is the lower-cost S&P Index tracker.
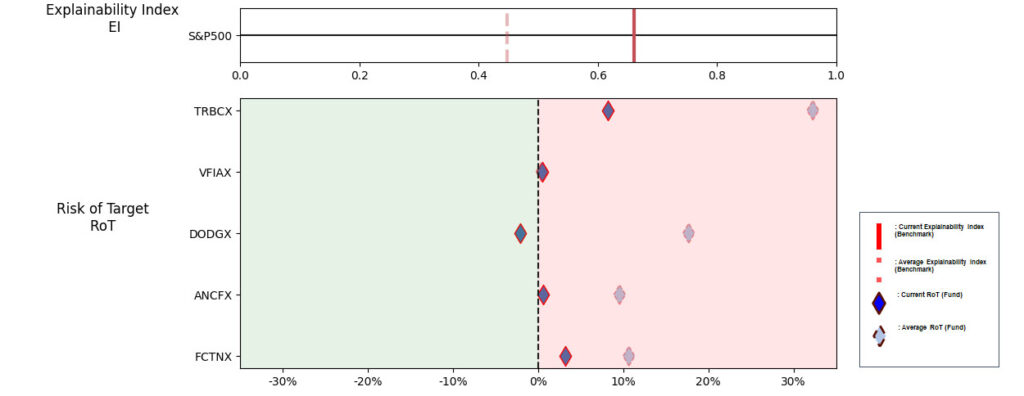
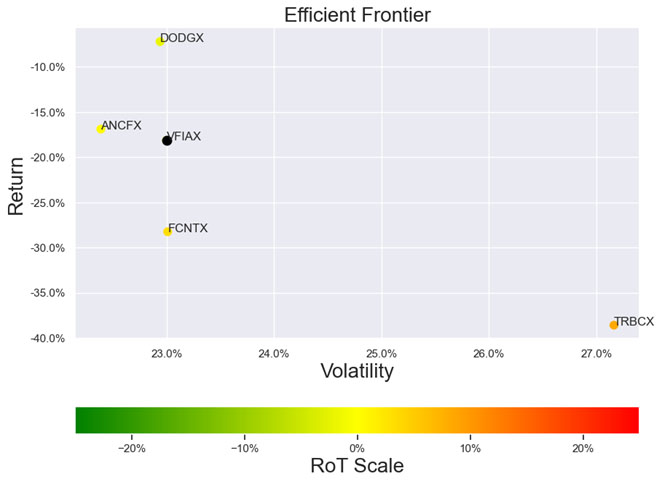
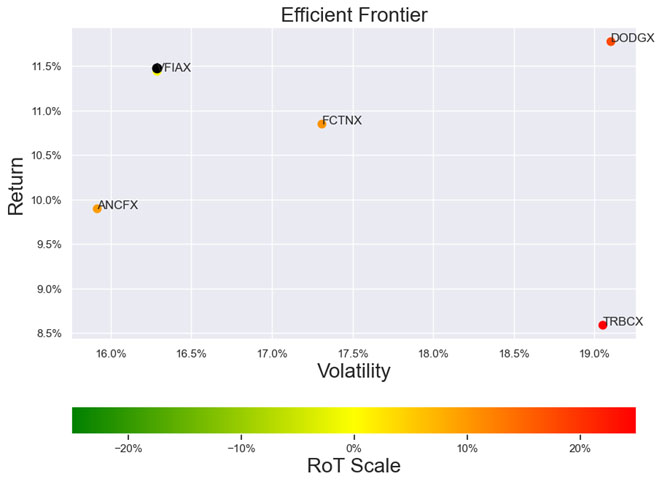
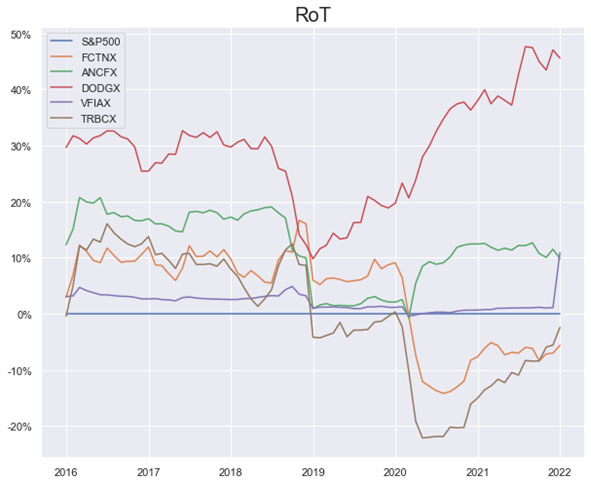
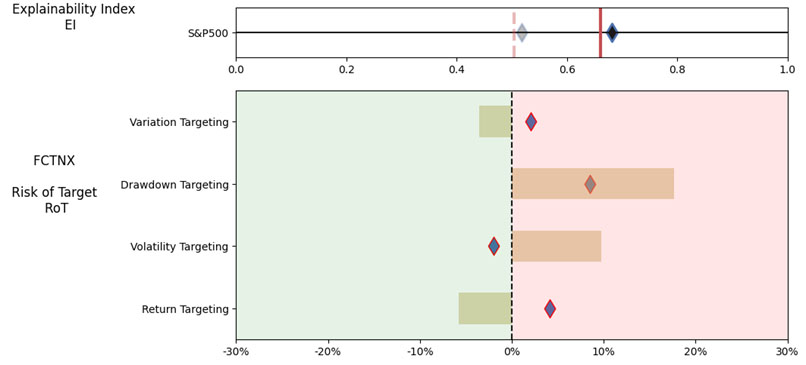
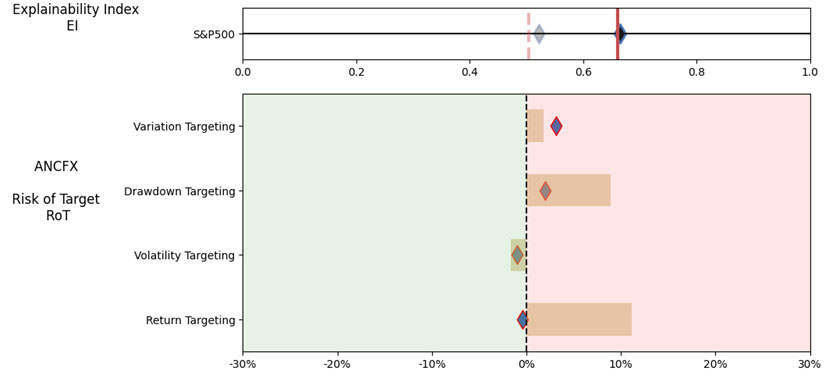
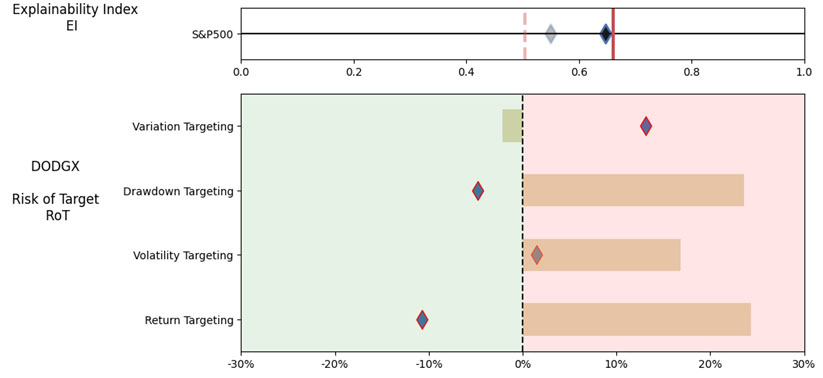
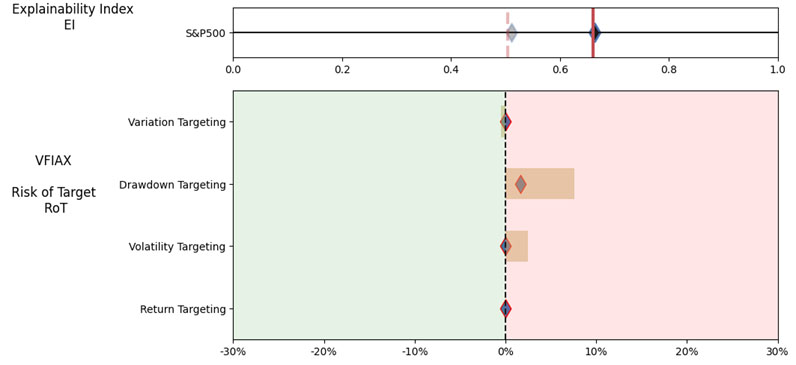
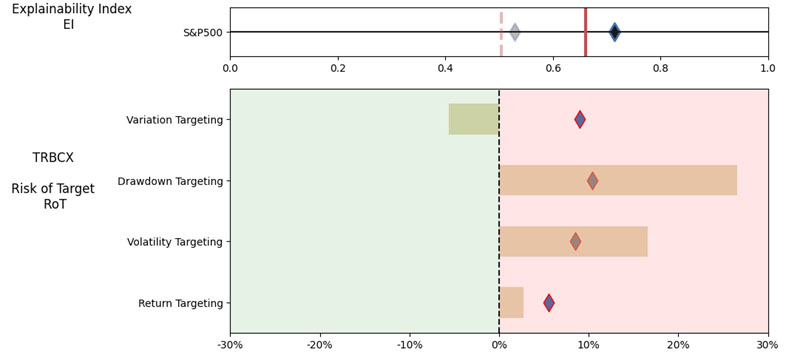
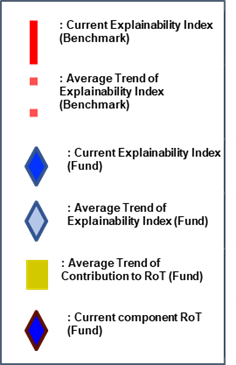
3.Best large cap US mutual fund (query on March 13th, 2023)
Our query was quite generic, where
- Location: US was specified
- Asset Class: Large Cap was specified
- Benchmark: None was specified.
As noted in Table 3. and Illustration 5. & 6., for the query ChatGPT suggested all US Large Cap funds and further the funds were from Blend, Growth and Value sub-classifications with expense ratios ranging from 0.02% – 0.66%. For our analysis, we assumed S&P 500 as the benchmark and two of the funds suggested were trackers and so including those the suggested funds had some potential choices in this set.
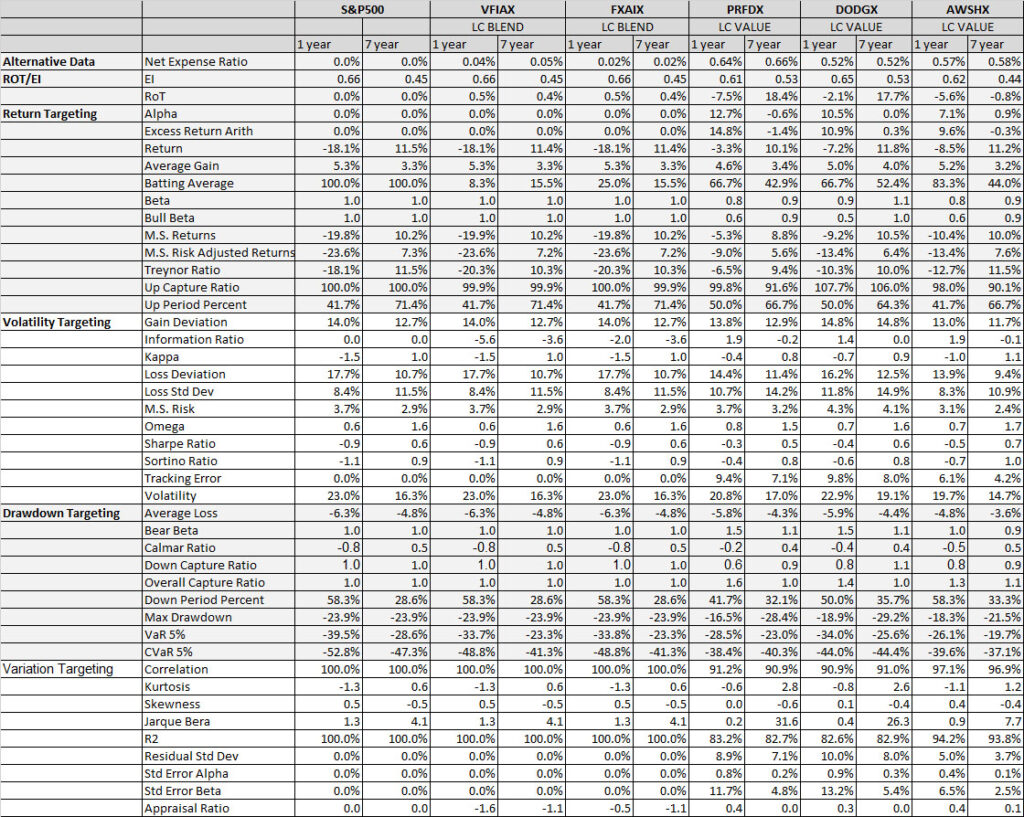
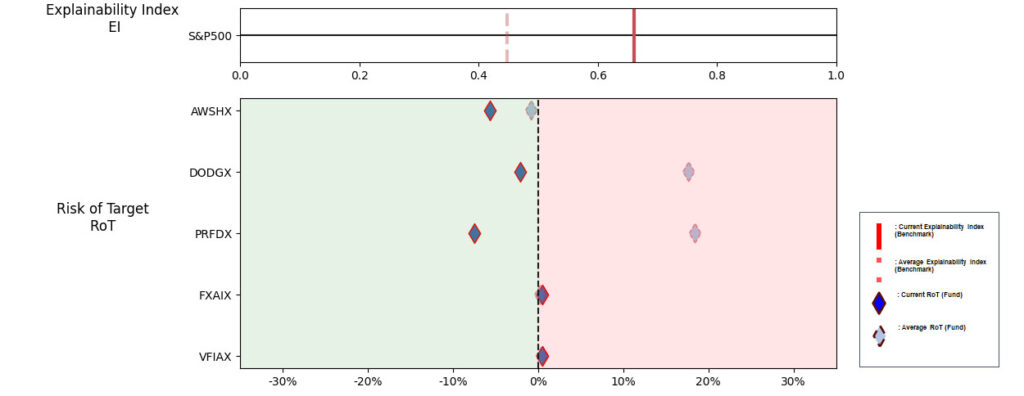
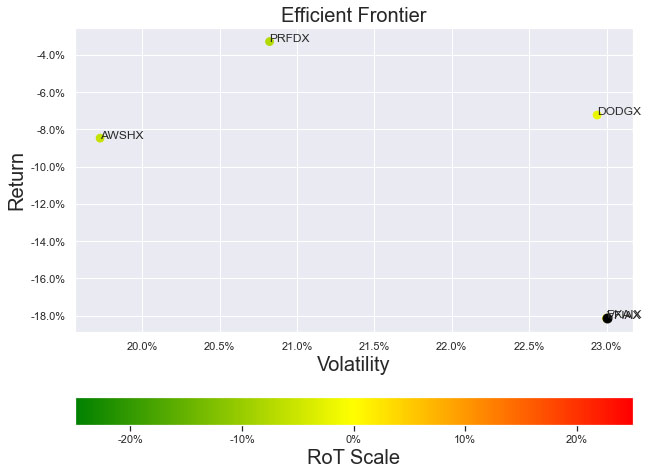
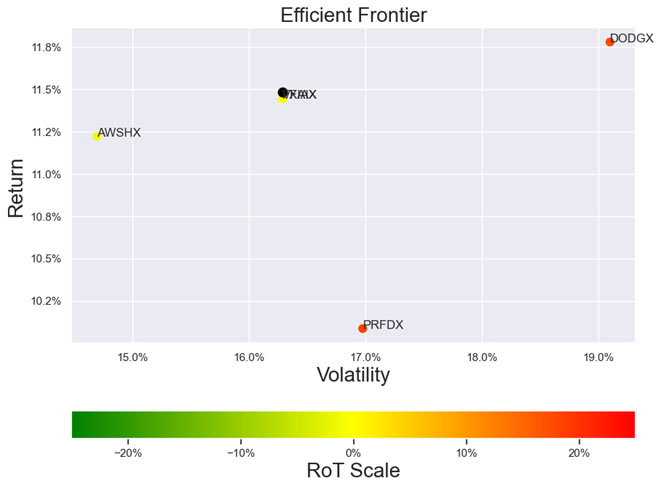
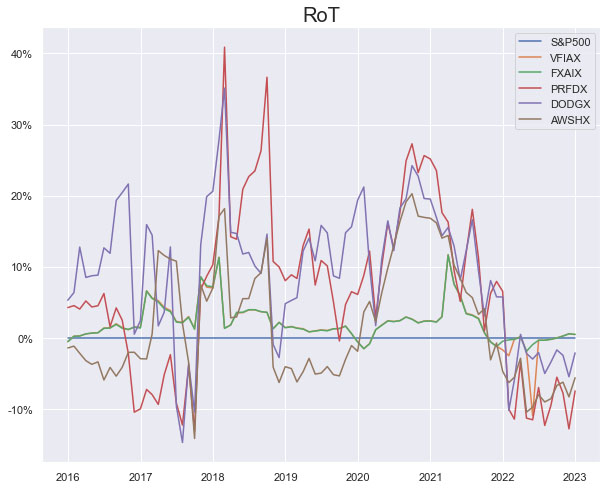
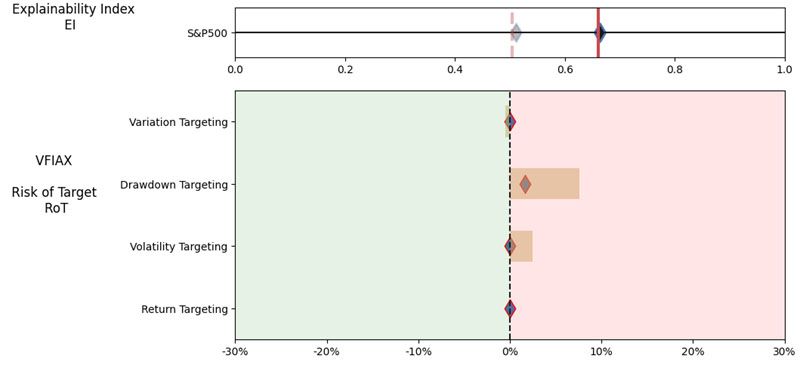
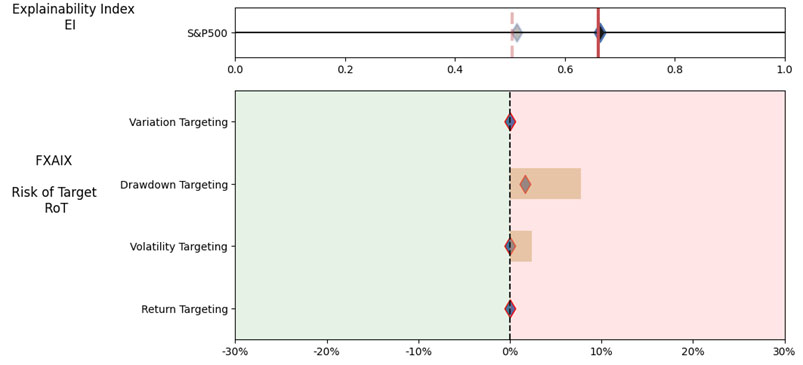
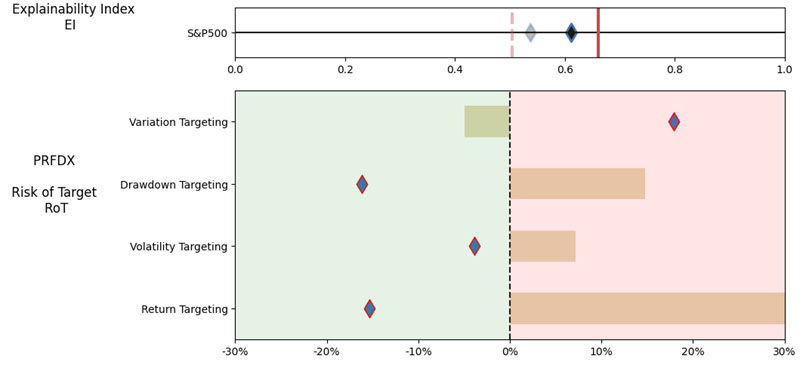
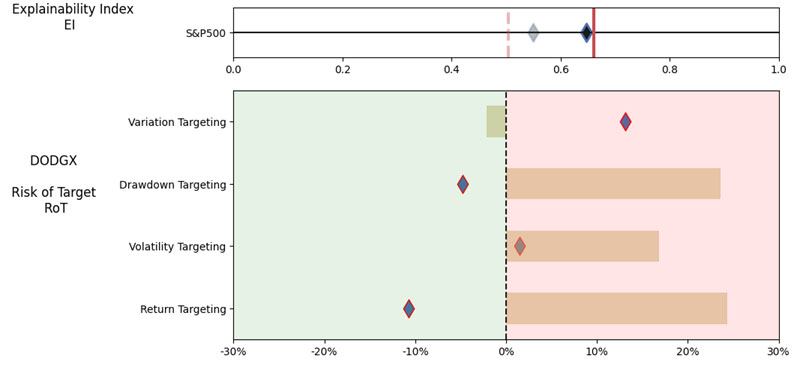
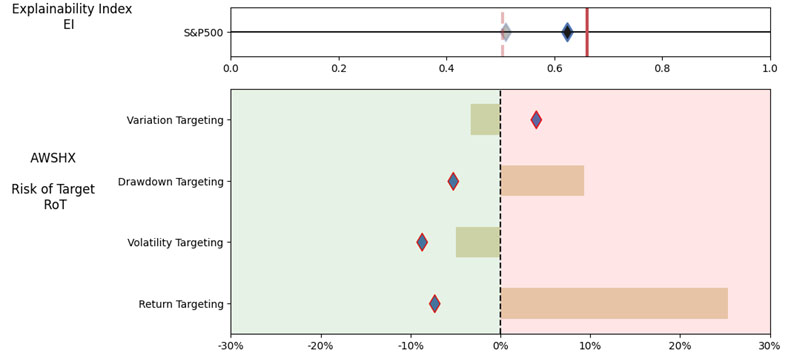
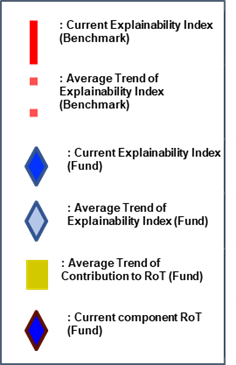
4. Best large cap US mutual fund (desktop query on March 13th, 2023)
Our query was quite generic, where
- Location: US was specified
- Asset Class: Large Cap was specified
- Benchmark: None was specified.
As noted in Table 4. and Illustration 7. & 8, for the query ChatGPT suggested US Large Cap funds (except potentially PRWCX) and further the funds were from Blend, Growth and Value sub-classifications with expense ratios ranging from 0.02% – 0.85%.
For our analysis, we assumed S&P 500 as the benchmark and three of the funds suggested were trackers and so excluding those the most of the suggested funds are questionable choices.
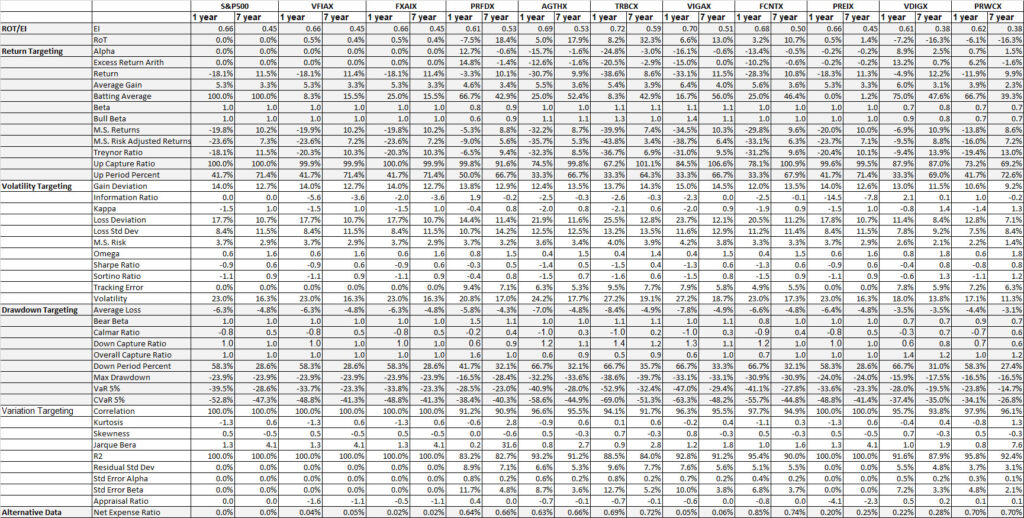
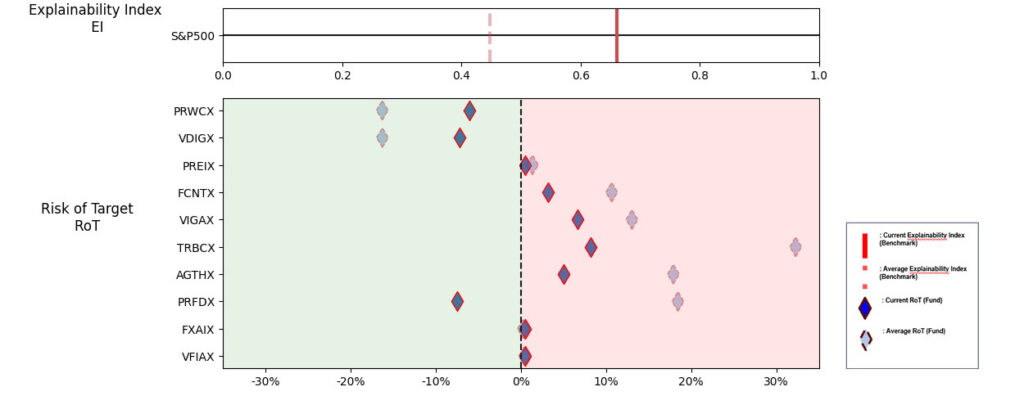
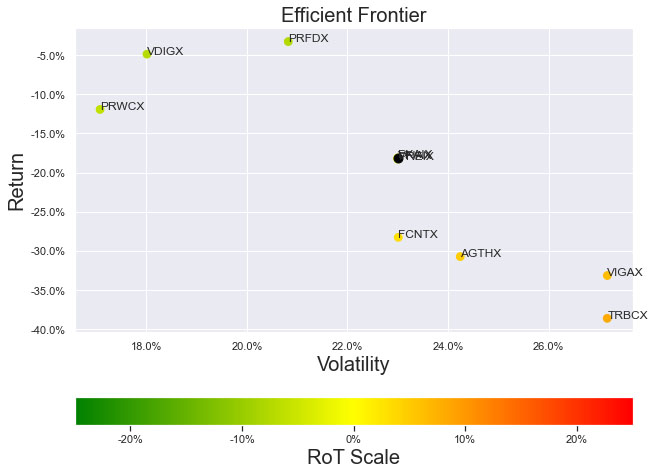
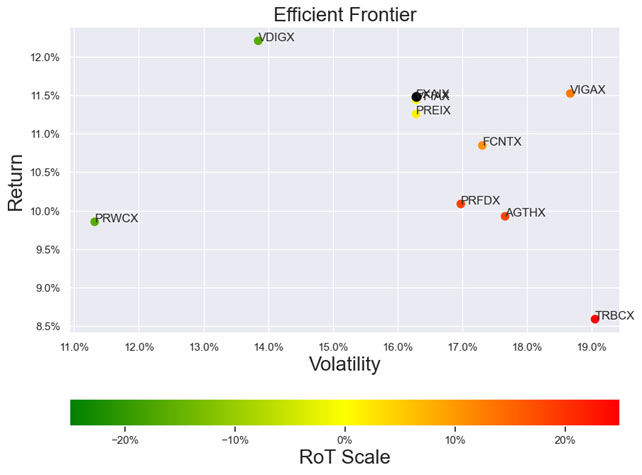
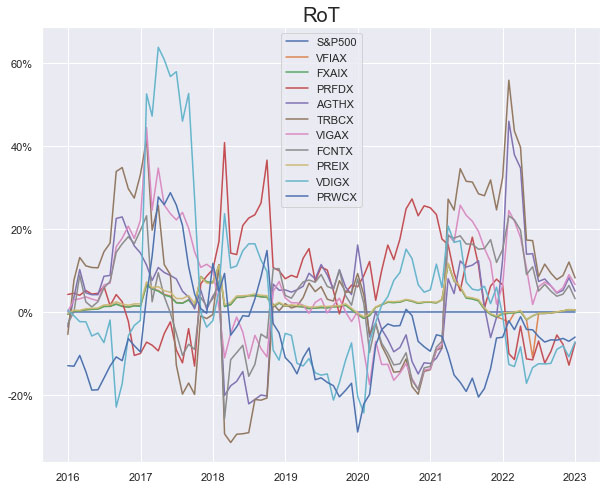
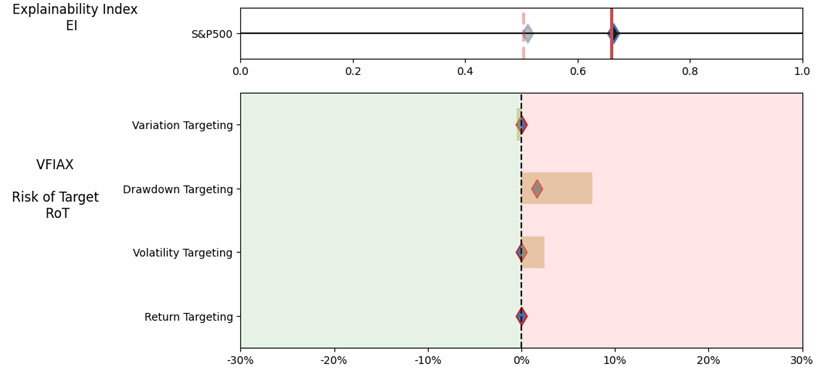
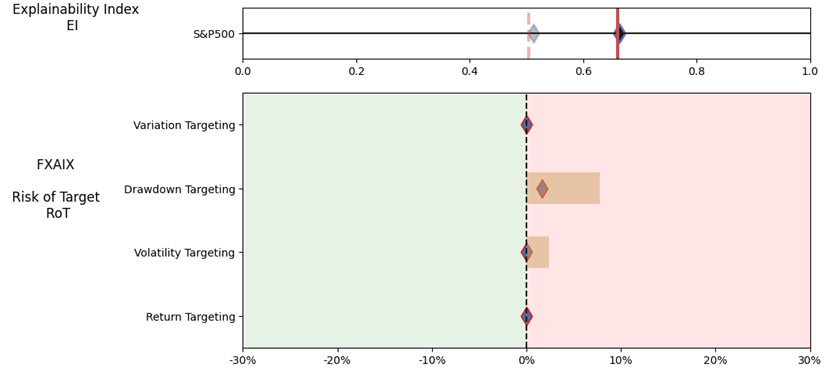
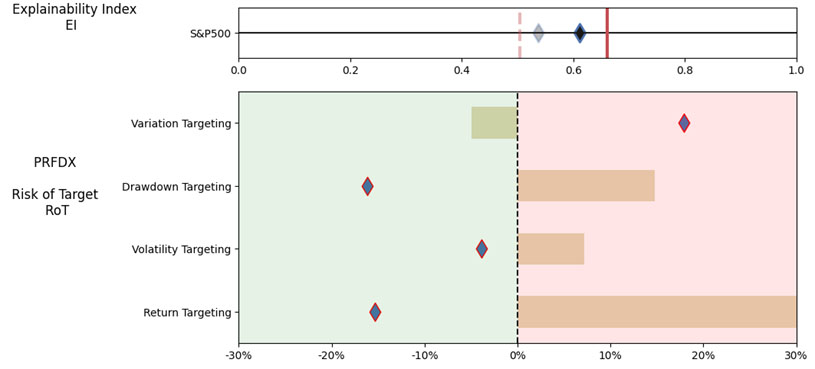
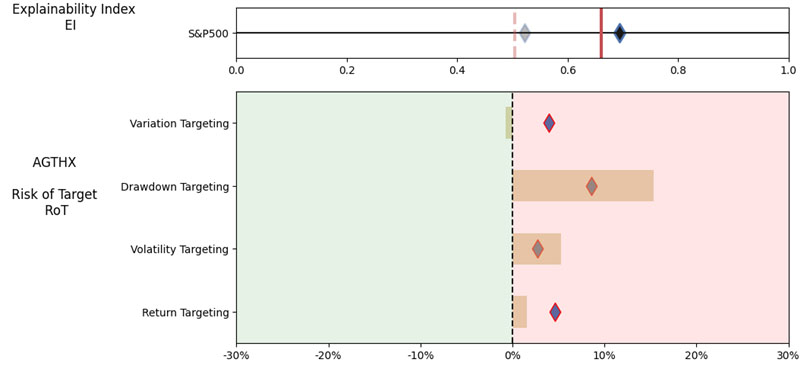
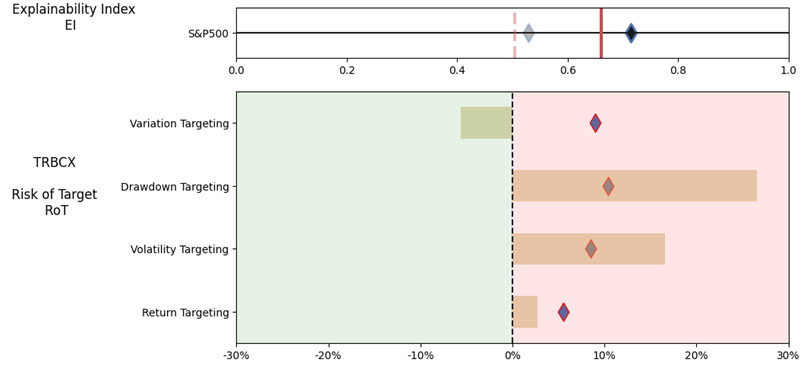
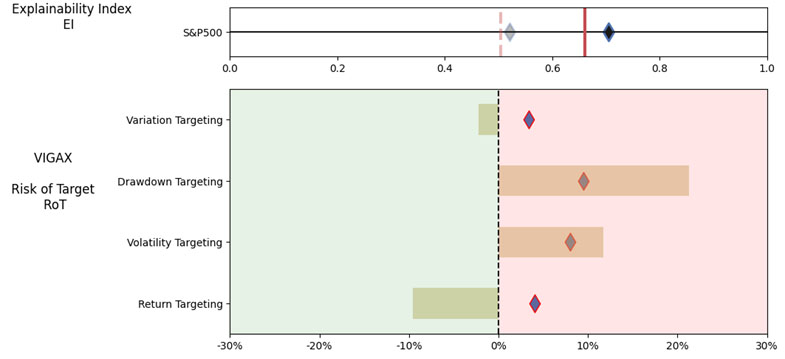
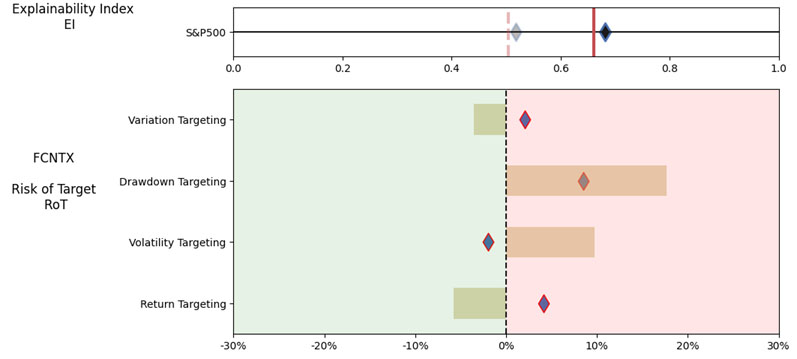
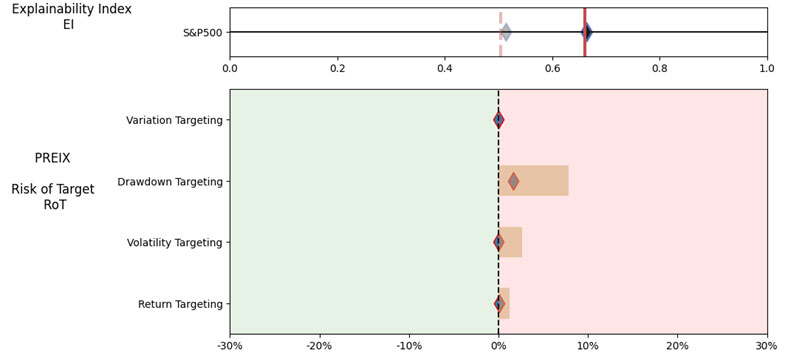
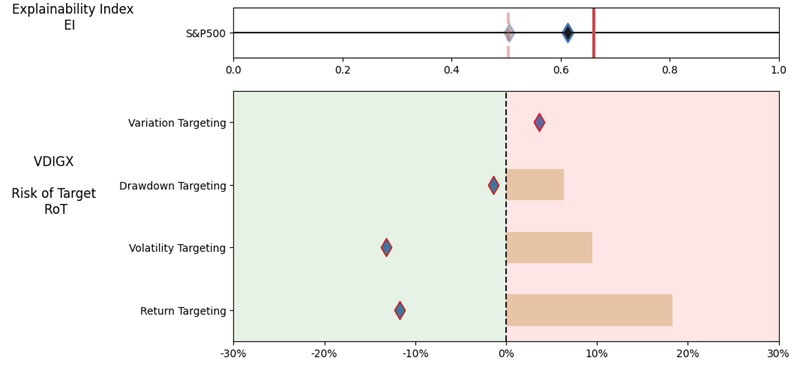
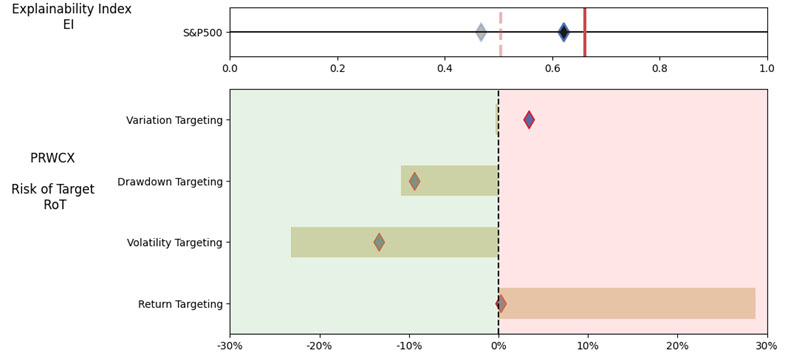
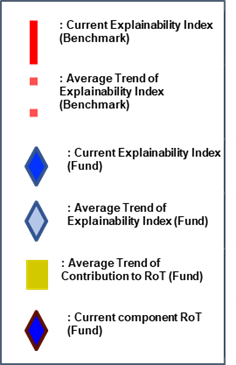
Overall, ChatGPT gave incomplete/inconsistent textual presentation and questionable fund suggestions especially as the reference benchmark was not specified. Further the results had cut-paste descriptions from the providers thereby potentially accentuating the biases. Overall, it remains a mystery as to why these were the results of the ‘best mutual funds” and/or “best large cap US mutual fund” queries, where one can only imagine that the LLM models found enough supporting language in the training dataset (e.g., offering documents, third-party write ups, etc).
Advisor beware – akin to patients visiting a doctor’s office armed with google reports, clients may visit your office armed with (annoying) ChatGPT suggestions!
Contact us for information about a particular fund, performance measure, time period, etc.
Email: info@ask2.ai for questions.
[1] At least in the current incarnation of the offerings. Financial markets are non-stationary and require tuning for each query.
[2] At the same time, setting guard rails on what information is good vs bad starts to infringe on the freedom aspects.
[3] 27,000+ if you assume all share classes. Also, not including SMAs, ETFs, etc.
[4] Hirsa, Ali and Ding, Rui and Malhotra, Satyan, Explainability Index (EI): Unifying Framework of Performance Measures and Risk of Target (RoT): Variability from Target EI (January 23, 2023). Available at SSRN: https://ssrn.com/abstract=4335455